5 minutes with Dr Lei Zhong: how we're building our credit engine
First things first: what is a credit risk decision engine?
A credit risk decision engine (RDE) is a set of algorithms that has just one job: to make decisions on risks, based on the probability of default over the next 12 months.
These algorithms operate in a very similar way to a human brain. When we have to make a decision, we consider the possible consequences and the probability of each of those outcomes. Then we choose the optimal option for us, based on the information we have at the time.
To put that into Bondaval terms: we are a financial provider, making decisions on credit risks. Before we issue a MicroBond, we need to make sure we are comfortable with the risk. Traditionally, this job has been done by human underwriters, and still is in many settings. These underwriters are financial experts, with years of experience, which allow them to recognize the telltale signs of a good or a bad company.
Our innovation at Bondaval is to use the machine learning method to:
- Drastically reduce the amount of time required to make human underwriting decisions.
- Increase the accuracy, or the predicting power, of all underwriting decisions.
External and internal data on human risk decisioning is fed into our credit engine, which then scores the risk from 1 to 100. Ultimately, this score determines whether we issue a MicroBond or not.
How do we build our credit risk models?
The core principles of the credit model are the same as those a human underwriter would apply. They might ask:
- What's the credit score of this company?
- What is the state of their financial accounts?
- Do they have any late payments, outstanding electricity bills or parking tickets, or any criminal records?
- How many accounts do they currently have open with other credit providers?
- What are the underwriting dynamics of the industry they operate in?
They will then combine this information to form an opinion.
Therefore, if we can understand how each data point contributes to the final decision, we can reflect that in our model, and use the machine to assess the company instead of asking a person to do it every time.
Building a model also means we can incorporate many more data points into our decision-making, without incurring the corresponding time-cost. The development process of the Bondaval Score considers thousands of data points for each company, across a number of different inputs.
For example, one, very powerful, additional input, alongside our other data sources, is the transaction history we can access through Open Banking.
A credit bureau report might be 12 months old, but with Open Banking, we can access the live transaction data of a company, going back 12 months. We can see how much money is going in and going out, along with the description for each transaction and whether it is corporate or personal.
The richness and freshness of this data means we can score the risk of a company defaulting far more accurately than ever before.
How does the underwriting team work with the credit model?
In the majority of cases, the answer of whether we should issue a bond is straightforward: a company might get a clear yes, or a clear no. For these companies, the RDE can run the underwriting algorithms very quickly, and return a decision in milliseconds.
Consequently, for these cases, underwriting can be fully automated via the credit engine. MicroBonds can then be issued automatically and at scale as a result, and human input is only necessary for monitoring and reviewing the decisions made by the machine.
There are a few exceptions, namely:
- Businesses with a complex company structure
- New geographies where data has not been fully collected
- Large security limits
In these exceptional cases, we still use the credit engine to provide an initial recommendation to our credit experts, then refer the case to them for a final credit review. This saves them from having to do everything from scratch.
There will always be some very complicated or niche cases that the machine will struggle to handle with a high enough degree of confidence. But as the machine processes more and more data over time and we refine our algorithms, the proportion of cases that need to be sent for further review will reduce drastically.
What makes our credit model different?
One of the major advantages of our model is the team behind it.
When it comes to modelling credit risk, people are traditionally from computer science and mathematics backgrounds (the world I come from). This is necessary for model building but it can sometimes mean that too much focus is put on the maths, to the detriment of including the underwriting team’s lived experience. This limits the effectiveness of the algorithms, as they might miss important signals that a human underwriter would spot instantly.
The Bondaval engine, however, combines expertise from a variety of backgrounds. Built on our market-leading mathematical and technological experience, the model also draws from the knowledge of our commercial team and advisors. This means that, together, we can develop our algorithms intelligently, allowing us to employ data as efficiently as possible and find patterns that inform both our decisions and the future of the Bondaval Risk Decisioning Engine.
The next stage, beyond reflecting human expertise in the model, is to feed results back into the machine, so that we can start to identify relationships between different patterns in the data and whether a company defaults or not. This may reveal correlations that aren’t immediately logical, but if we observe them frequently enough, may be important enough indicators of default to inform future iterations of the model.
Why did you want to join Bondaval?
I have more than 10 years of experience of building credit models and trying to use them to predict the behavior of businesses and their probability of default. Compared to other companies at a similar stage, Bondaval has achieved a lot already.
In terms of the technology, the fact that Bondaval is a new company is an advantage. I don’t have to spend time fighting legacy systems, or flaws in the model framework. I can spend my time building the best possible model and the best possible machine, using all my knowledge and experience. Bondaval also provides a fast feedback cycle, accelerating how long it takes for the model to upgrade.
Having said that, when I'm making the biggest decisions in my life, people are the most important factor. You need to be comfortable with the people you work with, have faith in their vision of the future and believe that the leadership is capable of steering a path to that vision. That’s the biggest reason I am excited to be here at Bondaval: the team and our collective vision for the future of credit.
The future of B2B credit risk protection
-
Non-cancellable coverage
-
Up to 100% balance sheet protection
-
Secured and adjusted digitally
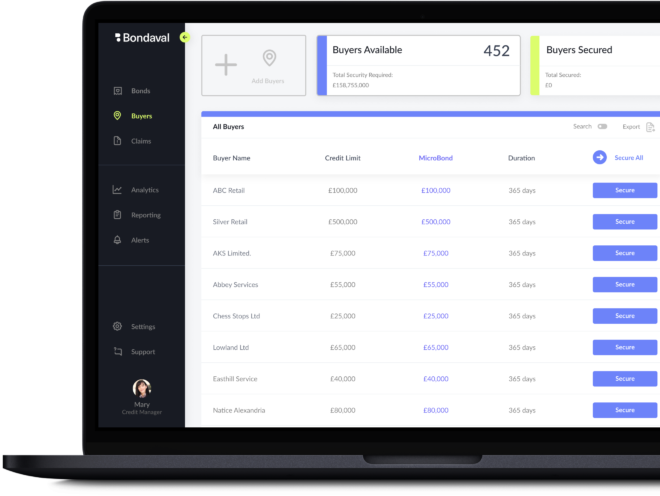